Context
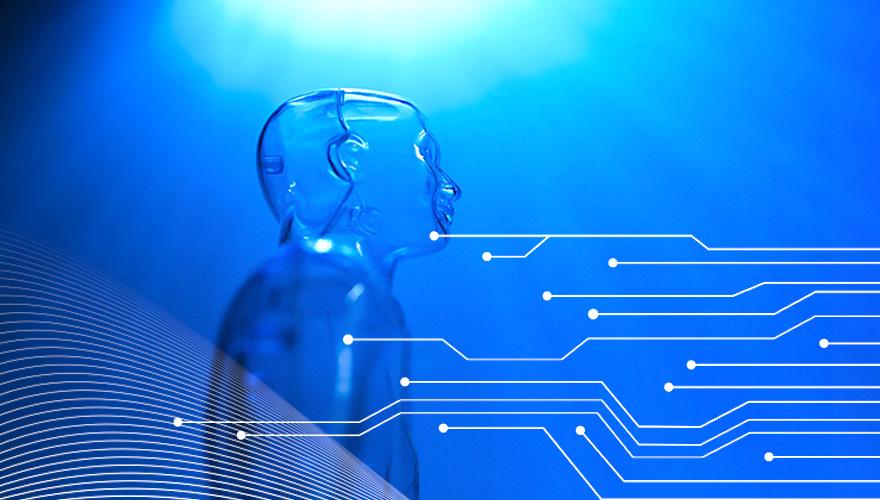
Artificial intelligence (AI) and machine learning (ML) capabilities are growing at an unprecedented rate. Countless AI applications are being or will be developed over the long term. In hindsight, one would say that progress certainly has taken place just looking at the range of tasks that AI and ML are able to solve autonomously today (according to the benchmarks) and were not solvable a few years ago, from machine translation to medical image analysis or self-driving vehicles. Moreover, progress in AI is widely believed to have substantial social and economic benefits, and possibly to create unprecedented challenges. In order to properly prepare policy initiatives for the arrival of such technologies, accurate forecasts and timelines are necessary to enable timely action among policy-makers and other stakeholders.
Approach
There is still much uncertainty over how to assess and monitor the state, development, uptake and impact of AI as a whole, including its future evolution, progress and benchmarking capabilities. While measuring the performance of state-of-the-art AI systems on narrow tasks is useful and fairly easy to do, in AI Watch we are trying to go one step further by mapping these performances onto more general AI. We aim to understand how these can have an impact on society in terms of benefits, risks, interactions, values, ethics, oversight into these systems, and more.
In brief, we are trying to provide a deeper understanding of the evolution and progress of AI by:
- Collecting, exploring, and monitoring data about AI results, progress and capabilities
- Analysing readiness and maturity levels of AI technologies
- Disentangling indicators, metrics, and explanatory factors behind AI results
Tools
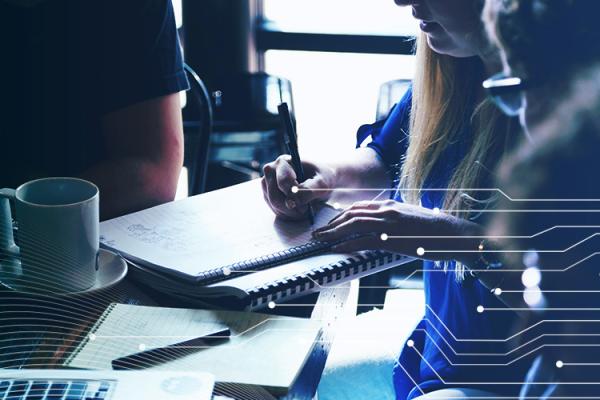
AI Collaboratory aims to develop a collaborative initiative for the analysis, evaluation, comparison and classification of AI systems.
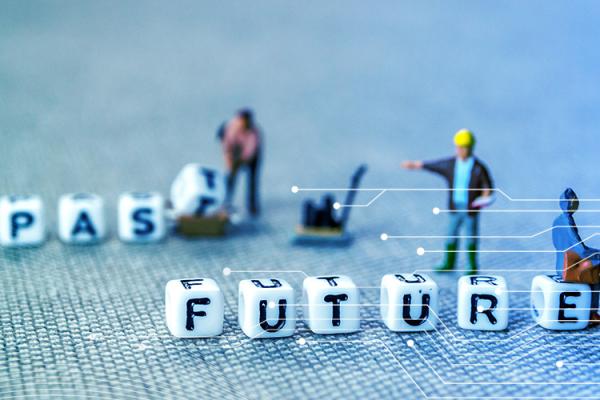
AI History Timeline
Publications
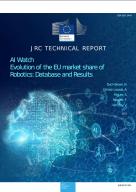
An overview of the robotics industry in Europe: definitions, typologies and differences between industrial and service robots.
This report proposes an example-based methodology to categorise and assess several AI technologies, by mapping them onto Technology Readiness Levels (TRL).
This report provides the analysis of multiple indicators related to the development of artificial intelligence from several perspectives.
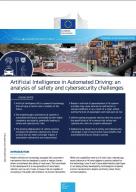
Addressing by design the AI safety and cybersecurity challenges is key to securing the many benefits that automated driving can bring to society.
The widespread use of experimental benchmarks in AI research has created competition and collaboration dynamics that are still poorly understood. This paper provides an innovative methodology to explore these dynamics and analyse the way different entrants in these challenges behave and react.
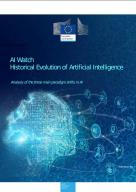
This report summarizes the evolution of AI, it introduces the “seasons” of AI development (i.e. winters for the decline and springs for the growth), describes the current rise of interest in AI, and concludes with the uncertainty on the future of AI.
News
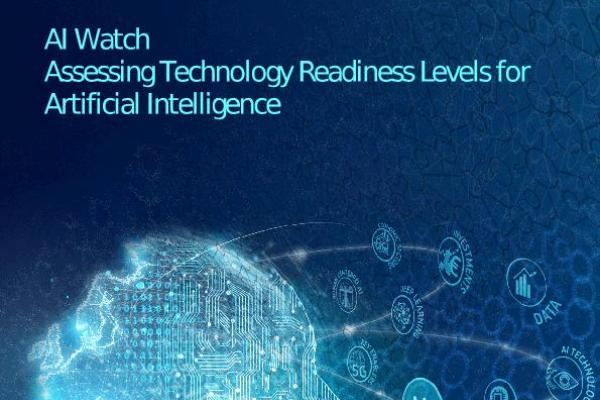
The new Joint Research Centre’s report “Assessing Technology Readiness Levels for Artificial Intelligence” aims to define the maturity of an illustrative set of AI technologies through the use of Technology Readiness Level (TRL) assessment.
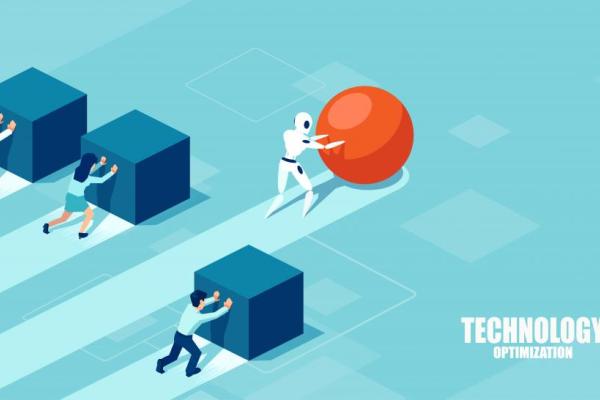
A recent study in Nature Machine Intelligence, analyses how benchmarking is transforming the Artificial Intelligence (AI) scientific research and its concrete applications in different fields.